UCLA and Amazon Announce Inaugural Recipients of Research Gifts and Amazon Fellowships
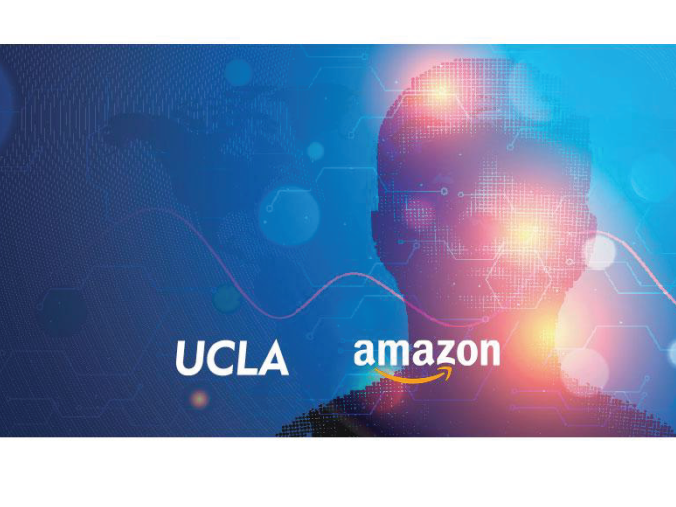
The UCLA Science Hub for Humanity and Artificial Intelligence, a collaboration with Amazon, announced today its first cohort of 12 Amazon fellowships and six gift-funded research projects.
Funded by Amazon and housed at the UCLA Samueli School of Engineering, the UCLA Science Hub was launched last October, marking Amazon’s first such alliance with a public university. The hub was established to facilitate synthesis between industry and academic research on artificial intelligence to address society’s most pressing challenges and develop solutions that will ultimately benefit humanity.
Twelve fellows were selected from a group of 25 UCLA Samueli doctoral students, and six research projects were chosen from 55 proposals by faculty across UCLA.
“I am excited to see the mission of the hub, harnessing the power of AI for the good of humanity, being carried out through collaborations among UCLA faculty, graduate students and Amazon scientists,” said UCLA Science Hub faculty director and computer science professor Jens Palsberg. “Together, we will break new ground in addressing the societal impact of AI and find real technological solutions to improve humanity.”
The Amazon fellows — doctoral students in computer science, electrical and computer engineering, and mechanical and aerospace engineering — will each receive up to two quarters of funding during the academic year to pursue independent research projects. They will also be invited to apply to intern at Amazon.
The inaugural cohort of UCLA Science Hub for Humanity and Artificial Intelligence Amazon Fellows and their research interests consist of:
Xiangning Chen
Automated and efficient machine learning, such as automatically identifying high-performance neural architectures and developing optimizers to accelerate large-scale pre-training
Advisor: Cho-Jui Hsieh
Ruchao Fan
Children’s automatic speech recognition (ASR) in a low-resource perspective and non-autoregressive end-to-end ASR models
Advisor: Abeer Alwan
Antonious Girgis
Communication-efficient and privacy-preserving machine learning as well as the trade-off between privacy and utility in statistical machine learning
Advisor: Suhas Diggavi
Ziniu Hu More efficient graph neural networks to model large-scale and complex graphs and differentiable symbolic reasoning with graph neural networks Advisor: Yizhou Sun | Zijie Huang Deep learning for reasoning over graph-structured dynamic data and modeling spatiotemporal data and knowledge graphs Advisor: Yizhou Sun and Wei Wang | Michael Kleinman Representation learning, computational neuroscience, machine learning and information theory, including usable information and evolution of optimal representations during training Advisor: Jonathan Kao |
Liunian Harold Li Learning vision-language alignment from unaligned data and using vision-language data to facilitate computer vision models Advisor: Kai-Wei Chang | Tao Meng Constrained inference for bridging the distributional gap in natural language processing and machine learning Advisor: Kai-Wei Chang | Yifan Qiao Democratization of large-scale machine learning training with full-stack systems and developing full-stack solutions covering ML algorithms and systems, operating systems and cloud infrastructures Advisor: Harry Xu and Miryung Kim |
Akash Deep Singh Bridging the gap between radio-frequency sensing hardware and machine-learning frameworks in mobile systems and the Internet of Things Advisor: Mani Srivastava | Weitong Zhang Optimization and machine learning, especially explainable and accountable reinforcement learning systems, and designing a provably data-efficient algorithm with application toward real-world problems Advisor: Quanquan Gu | Huajing Zhao Developing novel, multisensory-driven robotic systems with visual and tactile perception that enable dexterous manipulation control and decision-making for safe human-robot collaboration Advisor: Veronica Santos |
The recipients of six gift research awards will receive funding for exploratory projects in AI and machine learning. Four of the projects relate to potential applications of AI in addressing public health challenges:
RESEARCH PROJECTS
“Knowledge Graph Representation Learning and Applications in Biomedicine”
Wang will work to address some of the existing limitations that exist in most current knowledge graph models. This includes introducing a new way of deriving hierarchical knowledge graph representations, incorporating heterogeneous and dynamic propertiesthe , and creating a universal framework for real-world applications.
Wei Wang
Leonard Kleinrock Chair Professor in Computer Science
“Deep Learning for Biological Discovery with Application to Cardiometabolic Disease”
The research team will use deep learning-based processes and feature-attribution techniques to interpret the findings of the deep-learning techniques — all with the goal of making biomedical datasets as beneficial as possible to reduce burdens on public health.
Dr. Sriram Sankararaman Associate professor of computer science, human genetics and computational medicine | Dr. Paivi Pajukanta Professor of human genetics | Dr. Tzung Hsiai Cardiologist and professor of medicine and bioengineering |
“Prediction of Perinatal Depression Using EHR-Derived Phenotypes and Genetic Risk Scores”
Loohuis and Chiang will focus their project on developing a predictive model for depressive illnesses that occur during pregnancy or after childbirth. The project will use clinical and genetic predictors to develop a model to identify at-risk mothers, evaluate whether genetic risk scores can predict PND, and compare genetic risks for PND and non-PND depression.
Loes Olde Loohuis Assistant professor-in-residence of psychiatry and biobehavioral sciences | Jeffrey Chiang Assistant adjunct professor of computational medicine |
“Scalable Sequencing Approaches for Detection of Novel Pathogens and Evolving Viral Variants”
The group of researchers aims to expand the scope of SwabSeq — the scalable, inexpensive and accurate COVID-19 diagnostic PCR testing technology that is used across University of California campuses and beyond — to detect all known and unknown respiratory illnesses at scale.
Dr. Valerie Arboleda Pathologist and assistant professor of pathology & lab medicine, human genetics and computational medicine | Eleazar Eskin Professor and chair of Computational Medicine Department, and professor of computer science and human genetics | Chongyuan Luo Assistant professor of human genetics | Joshua Bloom Assistant adjunct professor of computational medicine | Leonid Kruglyak Distinguished professor of human genetics and biological chemistry |
The other two awarded projects focus on the application of AI and machine learning to address other societal needs:
“Fighting Wildfires with AI: Enabling High-Fidelity Wildfire Simulation Using Probabilistic Geospatial Deep Learning”
Taciroglu and Alipour will use artificial intelligence to advance wildfire management strategies, taking steps to mitigate the consequences of fire spread by creating real-time wildfire fuel maps to improve fire-spread simulation and response measures.
Ertugrul Taciroglu Professor and chair of Civil and Environmental Engineering Department | Mohamad Alipour Research assistant professor of civil and environmental engineering at University of Illinois Urbana-Champaign |
“Coresets for Efficient and Robust Machine Learning”
Mirzasoleiman will work to develop practical and theoretically rigorous methods to enable efficient and robust learning from massive datasets. The project will identify examples that provably contribute the most to learning and safely exclude those that are redundant or mislabeled.
Baharan Mirzasoleiman
Assistant professor of computer science